Easy statistics and bad science
Posted on 28 March 2017
Easy statistics and bad science
By Cyril Pernet, University of Edinburgh
I attended Brainhack Global in Warwick on 2nd and 3rd March 2017. On this occasion, I was invited to give a talk on reproducibility and listen to Pia Rothstein’s talk about pre-registration. These somewhat echo two papers published earlier this year: "Scanning the horizon: towards transparent and reproducible neuroimaging research" by Russ Poldrack et al. in Nature Neuroscience and "A manifesto for reproducible science" by Marcus Munafò et al. in Nature Human Behaviour. Here, I’ll talk about these two concepts that are 'new' because of the ease with which computers can nowadays generate huge quantities of statistical tests: analytical flexibility and full reporting.
Analytical flexibility vs. Pre-registration
Analytical flexibility refers to the many methods and software available to analyse a given data set. This 'degree of freedom' hinders reproducibility by (1) not being able to replicate the analysis workflow and (2) allowing p-hacking. Pre-registration is seen as one (among others) solution to this problem: register what analyses will be done in advance of data collection and stick to it, no matter if results are significant.
Munafo's analysis of the problem is particularly enlightening. We do an analysis (actually several) leading to a nice result that we can explain, in line with an hypothesis we make given such results. This is called p-hacking and harking. This happens not because researchers cheat but mostly because of confirmation bias: the tendency to interpret results as confirmation of one's existing beliefs. This implies that that we think that the analysis path that led to no good result was wrong, or there was a bug in the code whilst we do not question results that confirm our ideas (have you ever checked there is no bug on the code that gives the 'right' result?). Another cause of p-hacking & harking is hindsight bias: you think that the hypothesis you came up with after seeing the results could have been made in advance based on the literature alone. Again, this is likely not the case and you are fooling yourself. Pre-registration alleviates these natural, human biases by having to specify hypotheses, main outcomes, and the analysis path (or paths, nothing say you cannot pre-specify two analysis strategies) in advance, blinded from the data.
A typical argument of people who are against pre-registration is that if you specify it all in advance, you won't discover anything. I think this is missing the point: pre-registration allows to distinguish confirmatory from exploratory, not to prevent exploratory analyses. For instance, you can specify in advance that you are doing completely new research with no prior and that you will therefore perform N exploratory analyses on it. Although this is not what pre-registration in journal looks like, you can publish your pre-registered confirmatory analysis and add additional possible new effects based on total exploratory analyses. The difference is that you don’t pretend that the results were foreseen (and therefore you don’t know if you had enough statistical power for it), instead you present a possible discovery that needs replication.
Reporting
Poldrack et al. see the paper of the future as a software container (e.g. Docker) that runs the analysis code written using literate programming (and therefore produces the paper and figures). In theory, this is ideal for replicability: you write some code that reports the hypotheses you’ve pre-registered and execute it. Any additional analysis are presented as such, and new studies wanting to reproduce a discovery can just reuse the code. This also allows to see all the analyses performed in details and not hide any results (issues of selective reporting and publication bias).
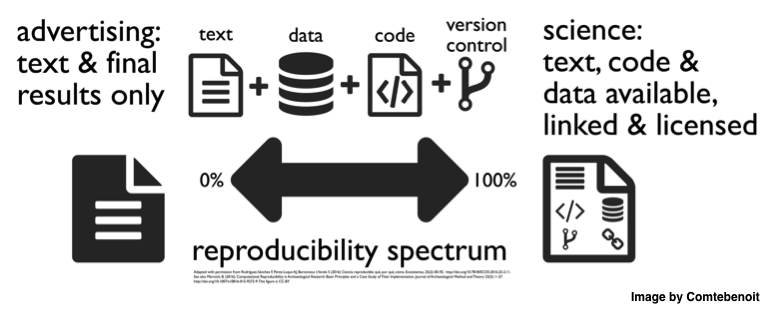
Unfortunately, it's not going to happen soon, unless the way science is done completely changes. Having a whole study contained and programmed will remain a step too far for many researchers. That is, to have such paper of the future, we will need larger multidisciplinary teams, including and recognising the role of research software engineers, and we’ll need to change how people are credited and evaluated. Then we may be able to see these papers of the future based on containers and run a code that produces the paper you just read.
Conference discussion
BrainHack is more like a workshop, unconference style. You typically have a good time discussing with people while working on different problems, and I certainly did have good discussions. Issues raised in this blog were not at the centre of such workshop, which was more about the cool tools in neuroimaging (details here). But a few of us discussed reproducibility—a problem which can be partly addressed with good software (How can study preregistration help neuroscience, Pia Rothstein, Birmingham, UK - Publishing a reproducible paper, Kirstie Whitaker, Cambridge, UK - Hacking the experimental method to avoid cognitive biases, Cyril Pernet, University of Edinburgh, UK - What the heck is wrong with brain research in psychiatry, Giovani Salum, Porto Alegre, Brazil - Preserving and Reproducing Research with ReproZip - Fernando Chirigati, New York, USA). I think it’s still not clear to many why we need to constrain ourselves on how we’re analysing the data and why we need to share them. This was certainly an on-going discussion in Warwick, where the workshop took place. I’m looking forward to the following hackday(s).